Learning Machines, Extended Logic, & Intelligence
This page contains loosely connected research lines on machine learning, information theory, as well as artificial and other intelligence. Learning Machines better reason according to logic. If uncertainties are involved, this should be extended, probabilistic, or Bayesian logic. The same is true for any form of intelligence, whether of human, artificial, or other nature.
Highlights
Manipulative communication in humans and machines
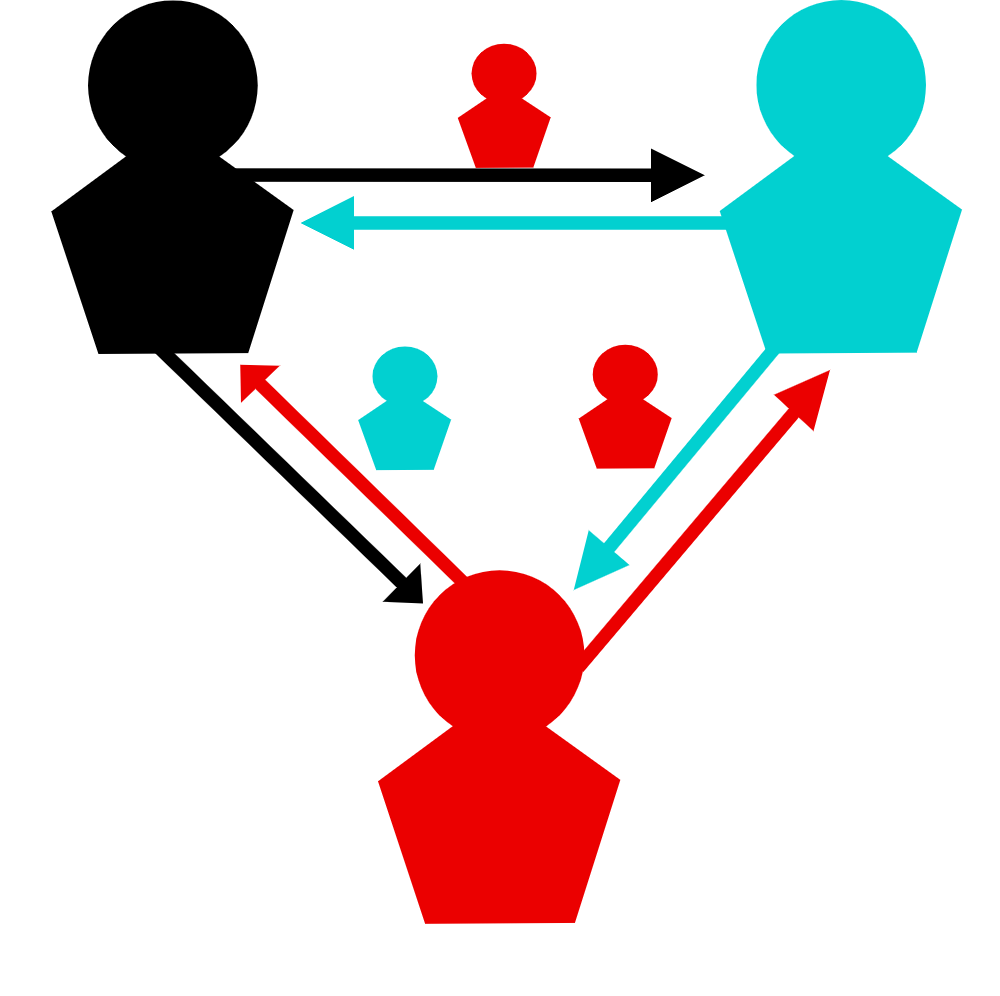
A universal sign of higher intelligence is communication. However, not all communications are well-intentioned. How can an intelligent system recognise the truthfulness of information and defend against attempts to deceive? How can a egoistic intelligence subvert such defences? What phenomena arise in the interplay of deception and defence? To answer such questions, researchers at the Max Planck Institute for Astrophysics in Garching, the University of Sydney and the Leibniz-Institut für Wissensmedien in Tübingen have studied the social interaction of artificial intelligences and observed very human behaviour.
- Learn more
- Learn more
Artificial intelligence combined
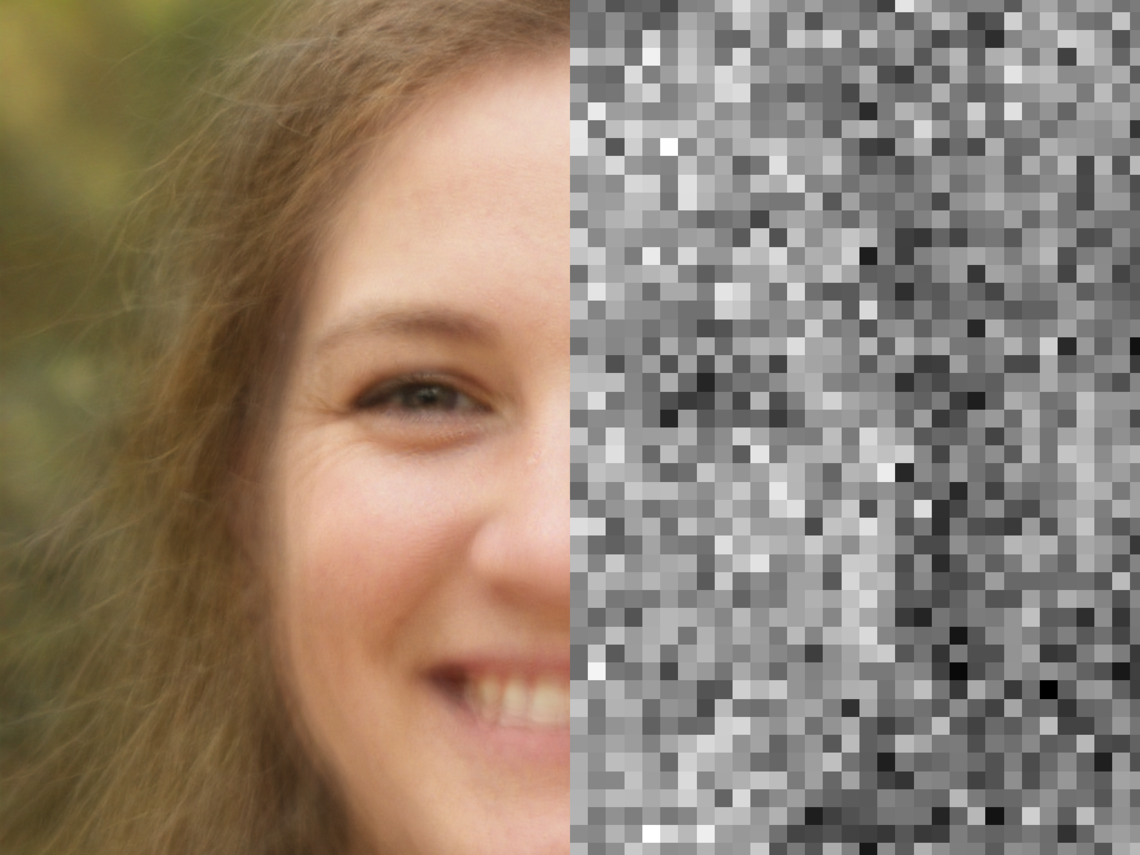
Artificial intelligence expands into all areas of the daily life, including research. Neural networks learn to solve complex tasks by training them on the basis of enormous amounts of examples. Researchers at the Max Planck Institute for Astrophysics in Garching have now succeeded in combining several networks, each one specializing in a different task, to jointly solve tasks using Bayesian logic in areas none was originally trained on. This enables the recycling of expensively trained networks and is an important step towards universally deductive artificial intelligence.
The embarrassment of false predictions -
How to best communicate probabilities?
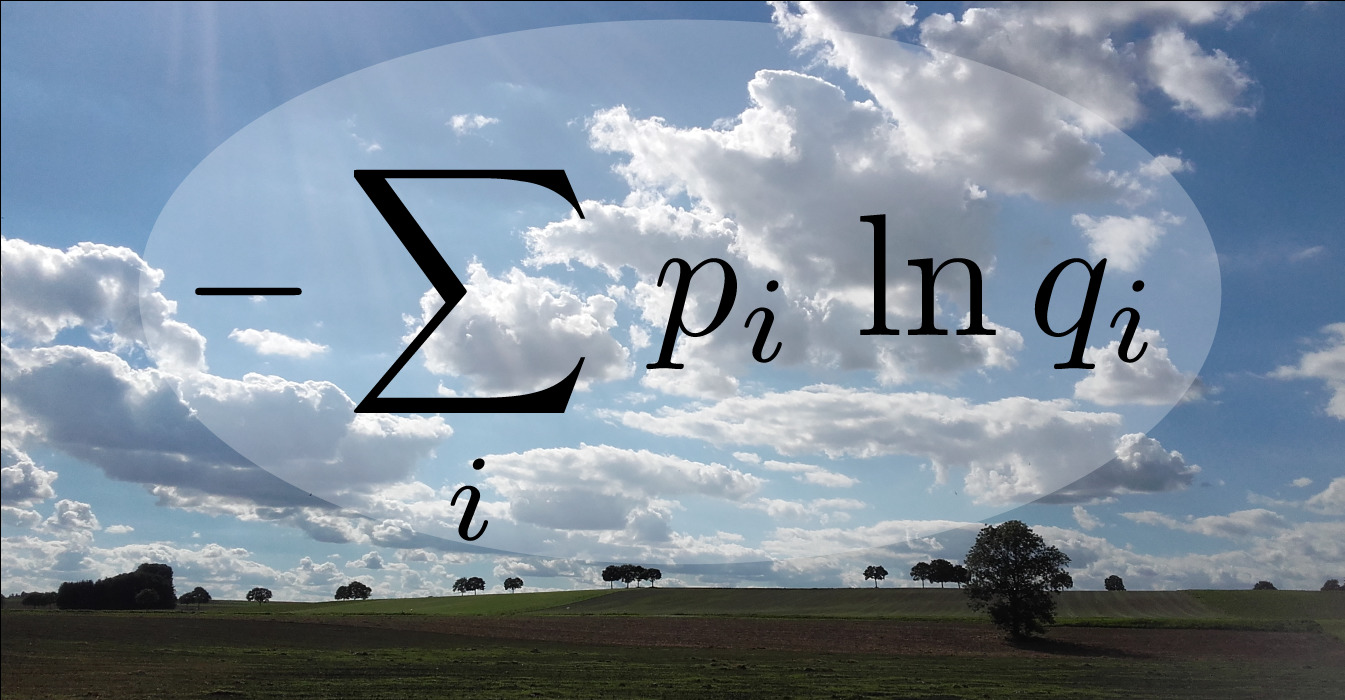
Complex predictions such as election forecasts or the weather reports often have to be simplified before communication. But how should one best simplify these predictions without facing embarrassment? In astronomical data analysis, researchers are also confronted with the problem of simplifying probabilities. Two researchers at the Max Planck Institute for Astrophysics now show that there is only one mathematically correct way to measure how embarrassing a simplified prediction can be. According to this, the recipient of a prediction should be deprived of the smallest possible amount of information.
Learning Machines
- Information Field Theory and Artificial Intelligence
Torsten A. Enßlin, Entropy 2022, 24, 374, arXiv:2105.10470 - Probabilistic Autoencoder using Fisher Information
Johannes Zacherl, Philipp Frank, Torsten A. Enßlin, 2021, submitted arXiv:2110.14947 - Geometric Variational Inference
Philipp Frank, Reimar Leike, Torsten A. Enßlin, 2021, Entropy, 23, 853, arXiv:2105.10470 - Bayesian Reasoning with Deep-Learned Knowledge
Jakob Knollmüller, Torsten A. Enßlin, 2021, Entropy 23, 693 arXiv:2001.11031 - Bayesian decomposition of the Galactic multi-frequency sky using probabilistic autoencoders
Sara Milosevic, Philipp Frank, Reimar H. Leike, Ancla Müller, Torsten A. Enßlin, A&A, 650, A100 arXiv:2009.06608 -
Information Field Theory: from astrophysical imaging to artificial intelligence
Talk by Torsten A. Enßlin at Joint Astronomical Colloquium (ESO Garching, 13.2.2020) - Sharpening up Galactic all-sky maps with complementary data. A machine learning approach
Ancla Müller, Moritz Hackstein, Maksim Greiner, Philipp Frank, Dominik J. Bomans, Ralf-Jürgen Dettmar, Torsten A. Enßlin, (2018) Astronomy & Astrophysics, Volume 620, id.A64, 20 pp. doi:10.1051/0004-6361/201833604 - Metric Gaussian Variational Inference
Jakob Knollmüller, Torsten A. Enßlin, submitted arXiv:1901.11033 - SOMBI: Bayesian identification of parameter relations in unstructured cosmological data
Philipp Frank, Jens Jasche, Torsten A. Enßlin, (2016) Astronomy & Astrophysics, Volume 595, id.A75, 18 pp. doi:10.1051/0004-6361/201628393 arXiv:1602.08497
Extended Logic
- A Bayesian Model for Bivariate Causal Inference
Maximilian Kurthen, Torsten A. Enßlin, Entropy 2020, 22, 46; doi:10.3390/e22010046 arXiv:1812.09895 - Encoding prior knowledge in the structure of the likelihood
Jakob Knollmüller, Torsten A. Enßlin, submitted arXiv:1812.04403 - Towards information optimal simulation of partial differential equations
Reimar H. Leike, Torsten A. Enßlin, Physical Review E, Volume 97, Issue 3, id.033314 ePrint, arXiv:1709.02859 - Optimal Belief Approximation
Reimar Leike, Torsten A. Enßlin, Entropy 2017, 19, 402; doi:10.3390/e19080402 arxiv:1610.09018 - Operator Calculus for Information Field Theory
Reimar Leike, Torsten A. Enßlin, Physical Review E, Volume 94, Issue 5, id.053306 (2016) arxiv:1605.00660 - Inference with minimal Gibbs free energy in information field theory
Torsten A. Enßlin, Cornelius Weig 2010, Physical Review E 82, 051112 arXiv:1004.2868 and Comment on Paper and Reply to Comment
Artificial, Human, & Other Intelligence
Torsten A. Enßlin, Annalen der Physik 2025, e2500057. https://doi.org/10.1002/andp.202500057, arXiv:2502.04088.
Viktoria Kainz, & Justin Sulik , Sonja Utz, Torsten A. Enßlin, submitted. https://osf.io/jdqc2
Fabian Sigler, Viktoria Kainz, Torsten Enßlin, Céline Boehm, and Sonja Utz. Phys. Sci. Forum 2023, 9(1), 3; https://doi.org/10.3390/psf2023009003.
Torsten A. Enßlin, Carolin Weidinger, Philipp Frank Annalen der Physik 2024, 2300334. https://doi.org/10.1002/andp.202300334; arXiv:2307.11423.
Viktoria Kainz, & Céline Bœhm, Sonja Utz, Torsten A. Enßlin, Entropy 2022, 24, 1768. https://doi.org/10.3390/e24121768
Viktoria Kainz, & Céline Bœhm, Sonja Utz, Torsten A. Enßlin, Phys. Sci. Forum 2022, 5(1), 39; https://doi.org/10.3390/psf2022005039. PsyArXiv:vd8w9
Torsten A. Enßlin, Viktoria Kainz, & Céline Bœhm, Physical Sciences Forum. Vol. 5. No. 1. Multidisciplinary Digital Publishing Institute, 2022. https://doi.org/10.3390/psf2022005015
Torsten A. Enßlin, Viktoria Kainz, & Céline Bœhm, Computational Communication Research, Volume 5, Issue 1, Jan 2023, p. 1. DOI:https://doi.org/10.5117/CCR2023.1.9.ENSS online article PsyArXiv:wqcmb
Torsten A. Enßlin, Viktoria Kainz, & Céline Bœhm, Annalen der Physik 2022, 2100277. https://doi.org/10.1002/andp.202100277, arxiv:2106.05414
Torsten A. Enßlin & Margret Westerkamp, Annalen der Physik, vol. 531, issue 3, p. 1800128 (2019) doi: 10.1002/andp.201800128 arxiv:1804.04948